The Rise of Vertical AI: A New Frontier
InfoThis is a summary of the following YouTube video:
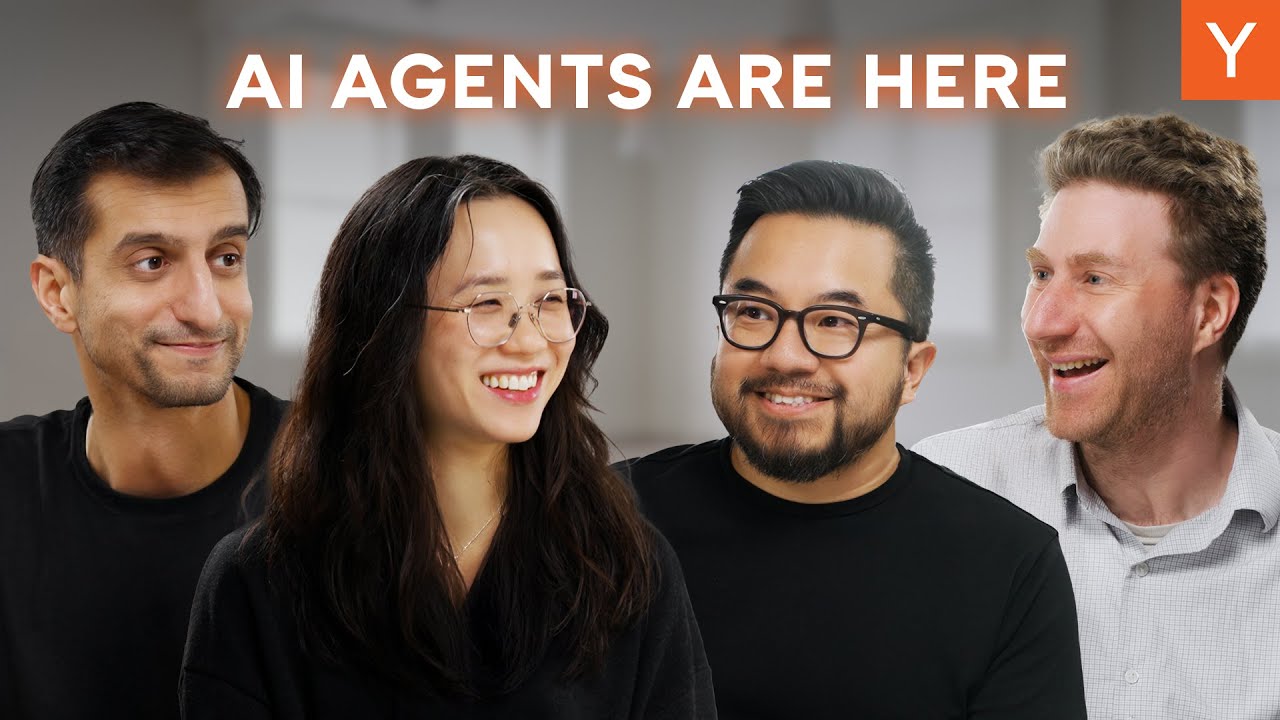
Vertical AI Agents Could Be 10X Bigger Than SaaS
Y Combinator
Nov 22, 2024
·
Science & Technology
Coming Up
- The text discusses the rapid advancement of AI models, highlighting the emergence of vertical AI agents that have the potential to replace entire teams and functions within enterprises. This progression is described as mind-blowing, indicating the significant impact these technologies could have on business operations.
- The conversation notes the increasing competition among foundational AI models, which were previously dominated by a single player, OpenAI. This shift towards a more competitive market is seen as beneficial, fostering a fertile marketplace ecosystem where consumers have more choices and founders have better opportunities.
- The hosts of the Lightcone podcast, including Gary, Jared Harge, and Diana, introduce themselves as experienced investors who have collectively funded startups worth hundreds of billions of dollars. This establishes their credibility and expertise in discussing the potential of vertical AI agents.
Jared is fired up about vertical AI agents
- Jared is enthusiastic about the potential of vertical AI agents, emphasizing their future impact on industries. He believes that many startup founders, especially younger ones, do not fully grasp the magnitude of this opportunity.
- Vertical AI agents are not a new concept, but their potential is not yet widely recognized. Jared predicts that there will be $300 billion companies emerging from this category alone.
- Jared draws a parallel between the rise of vertical AI agents and the historical growth of SaaS (Software as a Service) companies. He notes that SaaS has been a major focus for Silicon Valley, with over 40% of venture capital funding going to SaaS companies over the past 20 years.
- The introduction of XML HTTP request in 2004 was a catalyst for the SaaS boom, enabling the creation of rich internet applications like Google Maps and Gmail. This shift marked the transition from desktop software to web-based applications.
- Paul Graham was an early pioneer in SaaS, creating one of the first SaaS applications in 1995. However, early SaaS apps were limited by technology until the widespread adoption of XML HTTP request in 2005.
- Jared sees the current development of large language models (LLMs) as a similar paradigm shift, offering new opportunities for startups to create innovative solutions.
- He categorizes the paths to billion-dollar companies into three: obvious mass consumer products, unexpected mass consumer ideas, and B2B SaaS companies. The latter has produced the most billion-dollar companies.
- The lack of a dominant 'Microsoft of SaaS' has allowed for a diverse range of successful SaaS companies, as no single company can dominate all verticals and products.
- Salesforce is highlighted as the first true SaaS company, overcoming early skepticism about the viability of cloud-based enterprise applications.
- Jared compares the skepticism faced by early SaaS companies to current doubts about AI tools, suggesting that AI will similarly overcome these challenges and become integral to enterprise solutions.
The parallels between early SaaS and LLM’s
- The text discusses the potential for vertical AI agents to disrupt existing SaaS companies, drawing parallels to the early days of SaaS. Vertical AI agents are specialized AI models designed for specific industries or tasks, unlike general-purpose AI like voice assistants.
- The conversation highlights that while mass consumer applications, such as general-purpose AI voice assistants, present significant opportunities, they are likely to be dominated by incumbent tech giants. These companies have the resources and market presence to maintain their lead in these areas.
- The text references the 'innovator's dilemma,' where large companies may avoid pursuing risky innovations that could threaten their existing profitable operations. This is exemplified by companies like Google, which may hesitate to develop new products that could disrupt their core business, such as search.
- The discussion includes examples of how major companies like Google did not create clones of successful startups like Uber or Airbnb, despite their success. This reluctance is attributed to the potential regulatory risks and the threat to their existing revenue streams.
- The text mentions a talk by Travis Kalanick, co-founder of Uber, who expressed fears of personal legal risks during Uber's early years. This highlights the personal and financial risks entrepreneurs face when challenging established industries.
- Overall, the text suggests that while vertical AI agents have the potential to create new billion-dollar companies, they also face significant challenges from established tech giants and regulatory environments.
Why didn’t the big companies go into B2B SaaS?
- The discussion explores why large incumbent companies did not venture into B2B SaaS, highlighting the complexity and specialization required in each domain. Companies like Google may not have the expertise or interest in niche areas such as payroll, which are essential for products like Gusto.
- The conversation touches on the unbundling and bundling of software, explaining how vertical B2B SaaS products emerged as specialized solutions compared to broad, less user-friendly enterprise software from companies like Oracle or SAP.
- The shift from traditional software to SaaS and the internet allowed for more specialized and user-friendly solutions. Salesforce's success demonstrated that SaaS could be as powerful as traditional enterprise installations, paving the way for vertical SaaS solutions.
- Enterprise software often suffers from poor user experience because it tries to cover too much ground, leading to a 'jack of all trades, master of none' situation. Vertical B2B SaaS companies can offer significantly better user experiences by focusing on specific domains.
- The pricing structure in software is discussed, with three main price points: $5 per seat, $500 per seat, or $55,000 per seat, corresponding to consumer, SMB, or enterprise sales. Enterprise software is often chosen by high-level executives, not the end users, leading to suboptimal user experiences.
How employee counts might change
- The traditional model for startups and software companies has been that as revenue scales, the number of employees must also increase. This is evident in many unicorn companies today, which often have hundreds or thousands of employees as they reach significant revenue milestones.
- The advice given to startups is evolving. Previously, the focus was on hiring the smartest individuals for various roles like customer success or sales, and building large teams around them. Now, there is a shift towards hiring skilled software engineers who understand large language models (LLMs) to automate tasks and reduce the need for large teams.
- This shift suggests a change in how startups grow post-product market fit. By building LLM systems, companies can lower costs and reduce the need to hire large numbers of employees, potentially leading to future unicorn companies operating with very few staff.
- An example of this trend is seen in a company that used an engineer's mindset to handle marketing tasks, traditionally managed by a marketing team. This approach allowed the company to scale effectively with fewer people, demonstrating the potential of leveraging engineering skills in non-traditional areas.
- The use of LLMs and AI can provide even greater leverage than traditional software, allowing companies to achieve more with fewer resources. This could lead to a future where companies are able to operate efficiently with minimal staff, focusing on automation and smart engineering solutions.
The argument for more vertical AI unicorns
- The concept of vertical AI agents is compared to the evolution of SaaS companies, suggesting that for every SaaS unicorn, there could be a vertical AI equivalent. This is because SaaS companies often disrupted traditional software companies, and now vertical AI agents could disrupt SaaS companies by integrating software and human tasks into one product.
- Enterprises are currently uncertain about the specific AI agents they need. Experienced founders, like Brett Taylor, are creating platforms that allow enterprises to deploy custom AI agents. This approach is seen in companies like Vector Shift, which aims to help enterprises build internal LLM-powered agents using no-code or SDKs, despite enterprises not always knowing their exact needs.
- The history of SaaS shows that consumer applications paved the way for enterprise solutions. This pattern might not repeat with LLMs, as enterprises are already accustomed to point and vertical solutions, making them more open to adopting vertical AI agents without reverting to general-purpose platforms.
- The potential of vertical AI agents is highlighted by their ability to replace not only SaaS software but also reduce payroll expenses. Unlike SaaS, which requires operational teams, vertical AI agents can automate many tasks, leading to more efficient companies with fewer employees needed for data entry or approvals.
- The discussion suggests that vertical AI agents could be significantly larger than the SaaS companies they disrupt. This is because they offer a more comprehensive solution by integrating software and human tasks, potentially making them ten times as large as their SaaS counterparts.
Current examples of companies/uses
- Vertical AI agents are emerging as a significant trend, with companies like Outset using large language models (LLMs) to enhance survey and Qualtrics spaces. These agents aim to provide insights into customer needs by processing language data, targeting decision-makers in organizations to avoid job displacement fears.
- AI agents are transforming quality assurance (QA) testing by potentially replacing entire QA teams. Companies like MCH are leveraging AI to offer solutions that eliminate the need for traditional QA teams, focusing on engineering departments instead. This shift allows companies to scale without building large QA teams.
- In recruiting, AI tools are being developed to handle the entire recruitment process, reducing the need for human recruiters. Companies like Nico are creating software that performs technical and initial recruiter screenings, minimizing friction from recruitment teams worried about job loss.
- AI is also impacting developer support, with companies like Cap.AI creating chatbots that handle technical queries, reducing the need for large developer relations teams. These bots improve by ingesting documentation and past interactions, providing efficient support.
- The customer support sector is crowded with AI solutions, but few can replace complex workflows of large teams. Companies like Parel are developing sophisticated AI agents that can handle intricate customer support tasks, indicating a trend towards specialized solutions rather than general-purpose ones.
- The concept of hyper-specialization in AI tools is leading to the creation of multiple billion-dollar companies rather than a single dominant player. This is due to the need for tailored solutions that cater to specific industry requirements, as seen in customer support and other sectors.
- AI tools are extending managerial capabilities by processing vast amounts of information, potentially increasing the scale at which firms can operate. This is exemplified by Rippling's approach to integrating multiple SaaS verticals, allowing for efficient management and expansion.
- The integration of AI in business processes is likened to expanding the Dunbar number, enabling leaders to manage larger teams effectively. This is achieved through AI's ability to read and summarize vast amounts of data, enhancing decision-making and organizational efficiency.
AI voice calling companies
- AI voice calling companies are emerging as a significant subcategory within the AI industry, focusing on automating tasks traditionally performed by call center workers. This automation is particularly relevant in sectors like auto loan collections, where companies like Salient are making strides.
- Salient, an AI voice calling company, automates the process of contacting individuals about their auto loan payments. This task, often performed by low-wage workers in call centers, is characterized by high turnover and large staff requirements, making it ideal for AI automation.
- Salient has achieved high accuracy in its AI voice calling technology and has partnered with major banks, demonstrating the rapid advancement and adoption of AI in this field. The company has been operational since last year and has successfully integrated its services by selling top-down to large institutions.
- The AI voice infrastructure is supported by companies like Vapy, which enable quick deployment and scaling of voice applications. These companies have reached significant scale due to the excitement and potential of AI voice technology, which can be set up in a matter of hours.
- A key challenge for AI voice companies is retaining customers amidst competition from new technologies like OpenAI's voice APIs. Companies must continually innovate and raise the quality of their services to maintain customer loyalty.
- The evolution of AI applications, particularly in voice technology, has been rapid. Six months ago, AI voices were not realistic enough, but now they can effectively replace human callers. This progress is part of a broader trend of AI applications becoming more sophisticated and capable.
- The development of AI voice applications is part of a larger trend where AI is increasingly replacing entire teams and functions within enterprises. This rapid progression is unprecedented and highlights the transformative potential of AI technology.
- The competitive landscape for AI foundation models is expanding, with new contenders like Claude challenging established players like OpenAI. This competition fosters a dynamic market ecosystem, offering consumers more choices and opportunities for innovation.
What is the right vertical for you as a founder?
- The text discusses how aspiring startup founders can identify the right vertical for their AI ventures by focusing on boring, repetitive administrative tasks. These tasks are seen as potential areas for billion-dollar AI agent startups.
- It is suggested that founders should pursue areas where they have personal experience or a relationship, as this familiarity can lead to identifying promising opportunities.
- An example is given of a company called Sweet Spot, which is developing an AI agent to bid on government contracts. This idea originated from observing a friend's tedious job of refreshing a government website for new proposals.
- Another example involves a company that pivoted to create an AI agent for processing medical billing in dental clinics. This idea came from a founder's experience with his mother's dental practice, where he noticed the repetitive nature of processing claims.
- The text emphasizes the importance of identifying mundane tasks that can be automated by AI, suggesting that these areas hold significant potential for successful AI startups.
Outro
- The discussion focuses on the emergence of vertical AI agents, which are specialized AI models designed to perform specific tasks within particular industries. These agents are expected to significantly impact existing SaaS companies by offering more tailored solutions.
- The hosts draw parallels between the early days of SaaS (Software as a Service) and the current development of large language models (LLMs), suggesting that just as SaaS transformed business operations, vertical AI agents could revolutionize industry-specific applications.
- A key question raised is why major companies did not initially venture into B2B SaaS, hinting at potential opportunities for vertical AI agents to fill gaps in the market by addressing specific business needs.
- The conversation touches on how the adoption of vertical AI agents might alter employee roles and reduce headcounts, as these agents can automate routine and repetitive tasks, leading to more efficient operations.
- There is a strong argument presented for the rise of more vertical AI unicorns—startups valued at over a billion dollars—due to the increasing demand for specialized AI solutions that cater to niche markets.
- Current examples of companies utilizing vertical AI agents are discussed, highlighting their practical applications and the benefits they bring to various sectors, such as improved efficiency and cost savings.
- AI voice calling companies are mentioned as a specific example of vertical AI applications, showcasing how AI can enhance communication technologies by providing more personalized and efficient services.
- The hosts advise founders to carefully consider which verticals to target when developing AI solutions, emphasizing the importance of aligning AI capabilities with industry needs to maximize impact and profitability.
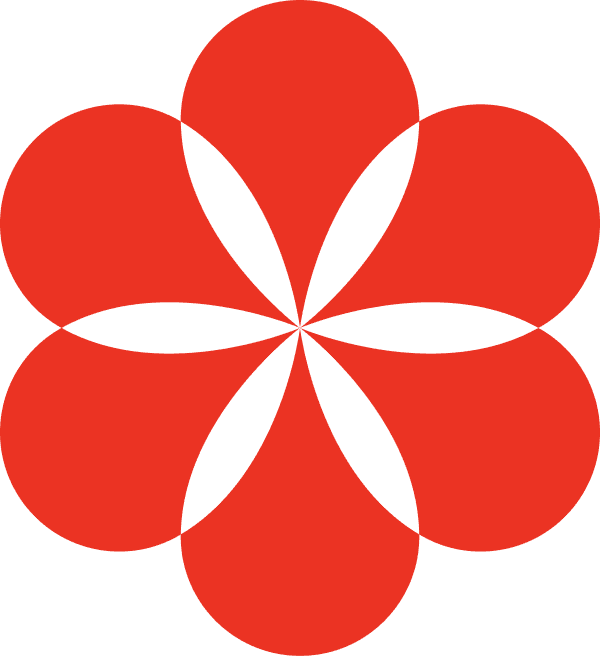
Turn any content into your knowledge base with AI
Supamind transforms any webpage or YouTube video into a knowledge base with AI-powered notes, summaries, mindmaps, and interactive chat for deeper insights.